Analyzing surgical technique in diverse open surgical videos with multi-task machine learning
2 Department of Biomedical Data Science, Stanford University; Stanford, CA, USA.
3 Department of Electrical Engineering, Stanford University, Stanford; CA, USA.
4 Clinical Excellence Research Center, Stanford University School of Medicine; Stanford, CA, USA.
5 Department of Surgery, Beth Israel Deaconess Medical Center; Boston, MA, USA.
6 Department of Biomedical Informatics, Harvard Medical School; Boston, MA, USA.
* These authors contributed equally to this work.
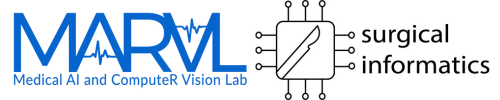
Links
Abstract
Importance: Globally, most surgeries are performed with open technique. Artificial intelligence (AI) has the potential to inform surgical training and optimize surgical care.
Objective:
- Overcome limitations of open surgery AI models by curating the largest collection of annotated videos
- Leverage this AI-ready dataset to develop a generalizable multi-task AI model capable of real-time understanding of clinically-significant surgical behaviors in prospectively collected real-world surgical videos.
Design: Programmatically queried open surgery procedures on YouTube and manually annotated selected videos to create the AI-ready dataset used to train a multi-task AI model for two proof-of-concept studies:
- Generating “surgical signatures” that define the patterns of a given procedure
- Identifying kinematics of hand motion that correlate with surgeon skill level and experience
Setting: The Annotated Videos of Open Surgery (AVOS) dataset includes 1997 videos from 23 open surgical procedure types uploaded to YouTube from 50 countries over the last 15 years. Prospectively recorded surgical videos were collected from a single tertiary care academic medical center.
Participants: Deidentified videos were recorded of surgeons performing open surgical procedures as well as simulated wound closure. Patients provided consent prior to their operation. The study was performed with Institutional Review Board approval.
Exposures: Our multi-task AI model was trained on the AVOS video dataset and then retrospectively applied to the prospectively collected video dataset.
Main Outcomes and Measures:
- Analysis of open surgical videos in near real-time,
- Performance on AVOS and prospectively collected videos,
- Quantification of surgeon skill.
Results: Using the AVOS dataset, we developed a multi-task AI model capable of real-time understanding of surgical behaviors—the building blocks of procedural flow and surgeon skill—across space and time. Through principal component analysis, we identified a single compound skill feature, composed of a linear combination of kinematic hand attributes. This feature was a significant discriminator between experienced surgeons and surgical trainees across 101 prospectively collected 97 surgical videos of 14 operators. For each unit increase in the compound feature value, the odds of the 98 operator being an experienced surgeon were 3.6 times higher (95% CI 1.67, 7.62, p=0.001).
Conclusions and Relevance: We directly applied our AVOS-trained model to analyze prospectively collected open surgical videos and identified kinematic descriptors of surgical skill related to efficiency of hand motion. The ability to provide AI-deduced insights into surgical structure and skill is valuable in optimizing surgical skill acquisition and ultimately improving surgical care.
AVOS (Annotated Videos of Open Surgery)
The AVOS dataset is a diverse, international dataset of publicly-available open surgical videos, filmed in a wide range of operating conditions. AVOS is comprised of 1,997 open surgical videos, each annotated with relevant United Medical Language System (UMLS) tags, approximately 48% of which are tagged with metadata, indicating that AVOS videos were uploaded from at least 50 countries throughout the last 15 years. Of these videos, 47 hours from 343 videos were further annotated temporally with surgical actions at a one second resolution, and spatially annotated with more than 12,000 tools and hands in addition to over 11,000 hand keypoints. Examples of the spatial and temporal annotations are shown below, along with each class label.
Temporal and Spatial Classification Demo
Open surgical videos contain complex and dynamic visual scenes relative to the more constrained visual content of minimally-invasive procedures and include intricate movements of multiple operators and tools, with significant variation in operative environments. This presents a unique challenge when training a multi-task neural network model that can perform simultaneous spatiotemporal analysis of hands, tools, and actions for open surgical videos. A demo of our network applied to an example video is shown below:
We are then able to leverage the model's inferences in order to illustrate the utility of our model in two proof-of-concept studies: 1) generating “surgical signatures” that define the patterns of a given procedure and 2) identifying kinematic features of surgical experience that distinguish experienced surgeons from surgical trainees using a dataset of unseen, real-world surgical videos collected at Beth Israel Deaconess Medical Center (BIDMC). This multi-task neural network represents a first essential step towards providing scalable, objective feedback of surgical skill without the bias of any individual surgeon’s judgement.
Citation
- title="{Analyzing surgical technique in diverse open surgical videos with multi-task machine learning}",
author={Goodman, Emmett D. and Patel, Krishna K. and Zhang, Yilun and Locke, William, and Kennedy, Chris J., and Mehrotra, Rohan, and Ren, Stephen, and Guan, Melody, and Zohar, Orr, and Downing, Maren, and Chen, Hao Wei, and Clark, Jevin Z., and Berrigan, Margaret T. and Brat, Gabriel A. and Yeung-Levy, Serena},
journal={JAMA Surgery},
year={2024},
month={02}
Webpage template from Tool Detection.