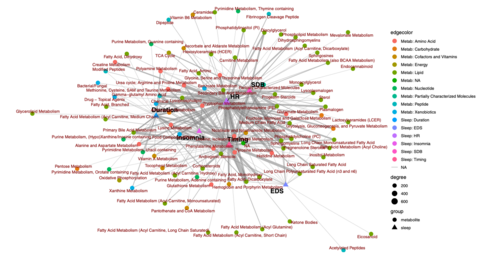
In our new published study we created an “atlas" of how sleep and metabolism are linked, using data from over 6,000 participants from the Hispanic Community Health Study/Study of Latinos (HCHS/SOL). In this analysis, we explored the relationships between 40 different sleep-related traits and over 700 metabolites in the blood. The goal was to better understand how sleep impacts our health, and create a resource that, ultimately, will help identify potential targets for new therapies.
The study looked at several aspects of sleep, including:
● Sleep disordered breathing (SDB)
● Sleep duration
● Sleep timing
● Insomnia symptoms
● Excessive daytime sleepiness (EDS)
● Heart rate during sleep
This study found that the link between sleep and metabolites varied across different metabolic pathways. For example, primary bile acid metabolism showed a high number of statistically significant associations with all sleep phenotypes except SDB and EDS. Several metabolites were associated with multiple sleep traits. For example, glycochenodeoxycholate, vanillyl mandelate (VMA), and 1-stearoyl-2-oleoyl-GPE (18:0/18:1) were linked to the highest number of sleep traits. Pregnenolone sulfate was associated with all sleep phenotype domains except sleep duration. N-lactoyl amino acids were associated with sleep duration, SDB, sleep timing and heart rate during sleep. Also, when we looked at groups of metabolites by superpathway, we saw that carbohydrates, cofactors and vitamins, lipids, and peptides were most associated with heart rate during sleep.
Individual metabolites in isolation were not strong biomarkers for sleep-related measures. Rather, complex patterns that can be better understood using network analysis. This analysis revealed that lipids and amino acids have a high degree of interconnectedness in how they relate to different aspects of sleep. In a network visualization, some metabolic pathways showed exclusive connections with one sleep domain, while others connected to multiple domains. This atlas provides a valuable resource for researchers, and highlights potential pathways for future studies.
We used notebookLM to prepare this summary (and then edited it). We also used notebookML to prepare a podcast, which you can listen to.
Audio file